Introduction
Big data has transformed industries by enabling creative solutions across sectors and offering profound insights. These sectors include, for example, marketing, finance, and precision medicine. However, many industries also use data that exposes every facet of peoples’ lives, which are very private. These highly personal facts include internet activity and health history. As a result, ethical challenges of big data concerning its gathering, application, and preservation have surfaced. Algorithmic prejudice, data security breaches, and privacy infringement have also become serious problems. As a result, tackling these issues calls for developing new technologies and moral principles backed by laws. This article examines major ethical issues related to big data and the obstacles certain industries face. It also explores best practices for ethically managing big data.
Section 1: Data Privacy and the Ethical Challenges of Big Data
Subsection 1.1: Informed Consent and the Ethical Challenges of Big Data
Many industries collect and use personal information to deliver highly beneficial goods and services. However, many organizations have highly publicized cases where they use personal data without individuals’ consent. Individuals have the right to know how organizations collect and use their data. Consequently, regulators must define clear standards for obtaining and managing consent. However, obtaining consent is challenging since consent forms are often unclear and filled with jargon. Several technological solutions, including blockchains and machine learning, promise to alleviate this problem. Blockchain can provide secure and consent mechanisms, while machine learning can anonymize data and retain utility.
Subsection 1.2: Data Minimization and the Ethical Challenges of Big Data
Like the least privilege concept in cybersecurity, companies shouldn’t collect more data than they need. Thus, data minimization will lower the chance of breaches, yet it depends on feasible organizational and technological solutions. Regulations and industry-wide rules that do away with pointless data collecting and buildup are examples of organizational solutions. A technical approach that narrows the data collection area is using predictive analytics tools. Training artificial intelligence (AI) algorithms to function well with little data input is another technical approach. Privacy-preserving technology employs differential privacy—a technical method for addressing the ethical challenges of big data while protecting user identity.
Subsection 1.3: Anonymization and Pseudonymization
Anonymization and pseudonymization of personal data are additional ways businesses can uphold individual privacy and lower privacy risks. New developments in cryptography have made stronger anonymization approaches possible. Machine learning methods should, however, take re-identification concerns into account. Businesses can use these methods to enable safe multi-party computation that permits analysis without requiring access to raw data. Regulators could also mandate the adoption of pseudonymization for companies operating in high-risk sectors.
Subsection 1.4: Cross-Border Data Transfers
Many companies operate across borders where different countries have varying data privacy and sharing regulations. This also applies to companies doing business with companies in other countries. Therefore, most companies face challenges in complying with multiple privacy laws. Consequently, organizations must carefully manage international data flows to avoid privacy breaches. However, several technological solutions can assist organizations. Encryption technology can ensure secure international data exchanges. Also, AI systems can incorporate regional compliance mechanisms to adhere to regulations.
Subsection 1.5: Right to Be Forgotten
Individuals have a moral and increasingly legal right to request that companies delete their data from company records. Regulatory bodies are also increasingly emphasizing the need for effective data deletion mechanisms. Afterward, companies must simplify the processes that handle “right to be forgotten” requests. Therefore, they must build technologies that allow for easy retrieval and erasure of data upon request. They must also design AI systems to forget data that is no longer necessary.
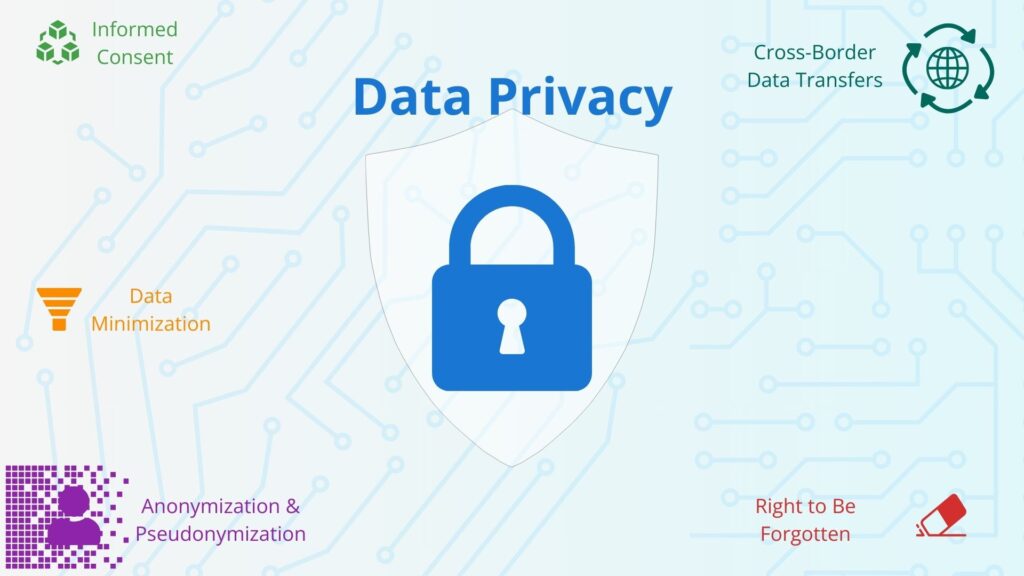
Section 2: Ethical Challenges of Big Data in Data Security – Safeguarding Sensitive Information
When companies handle personal information, they assume responsibility for its protection. Also, regulatory authorities legally enforce data protection on companies. Therefore, companies must protect data using both personnel and technical means.
Subsection 2.1: Encryption
Companies must encrypt all data stored and transmitted. Engineers use the terms “at rest” and “in transit,” respectively. Encryption is the first defense against unauthorized access, and all companies should adopt end-to-end encryption. Companies must also use established robust encryption algorithms and never use homegrown algorithms. Additionally, companies must develop AI and machine learning models to work with encrypted data. Furthermore, companies must stay ahead of the curve with the advent of quantum computers threatening to upend existing encryption algorithms. Therefore, companies must conduct regular audits that ensure encryption standards remain effective.
Subsection 2.2: Access Control
Companies must also implement personnel policies as part of their response to data protection. Implementing the least privilege principle will limit access to data and reduce the risk of insider threats and data breaches. Companies implement least privilege through role-based access control, ensuring only authorized personnel can access specific data sets. Also, companies should regularly update these access controls to match current security standards. Additionally, it is now mandatory to implement multi-factor authentication with biometrics because threat actors are now far more sophisticated. Also, companies need AI-driven systems to help monitor and manage access points in real time.
Subsection 2.3: Secure Data Storage
Companies must implement best practices for secure data storage as an indispensable step in data protection. These best practices begin with encryption “at rest” and implementing backup and recovery systems. Next, companies must regularly apply security patches to storage systems as the threat environment evolves. Modern cloud storage systems offer scalable and secure data storage capabilities. Additionally, AI systems can now detect potential vulnerabilities in storage infrastructures.
Subsection 2.4: Ethical Challenges of Big Data in Threat Detection and Response
Companies must proactively protect their data and not only react to breaches. This includes early security threat detection to prevent large-scale breaches. Companies must also implement effective response mechanisms upon security breach occurrence. They should automate response systems to reduce the time needed to counter security threats. Proactive security measures include machine learning algorithms that analyze vast data streams to detect anomalies.
Additionally, AI-driven analytics can predict and identify potential attack vectors. Companies must augment preventative measures with effective processes, policies, and employee training. Overall, companies must invest in threat intelligence systems for comprehensive security.
Subsection 2.5: Data Breach Protocols
Companies must implement effective data protection, including both personnel and technical protocols. Subsequently, they must implement plans, policies, and processes for data breach response, including employee training. They must also build a cyber security culture. Technical solutions include AI systems that can automate breach detection and alert key stakeholders. Another technology is blockchain, which provides a transparent, immutable audit trail. Companies must regularly test and update breach response plans to remain effective. Companies must also conduct post-mortems whenever breaches occur to adopt lessons learned to improve security protocols.
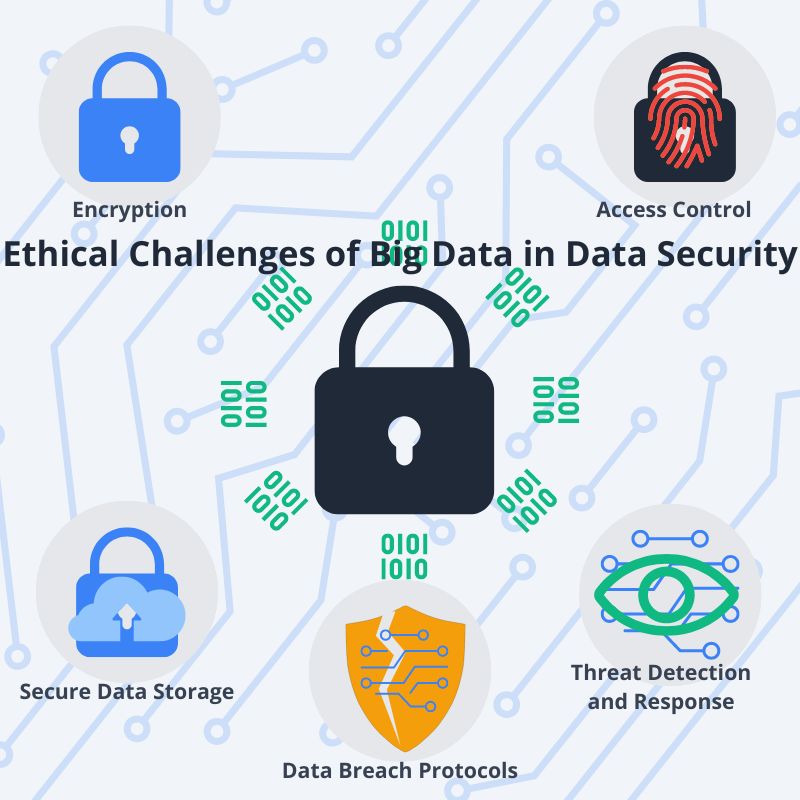
Section 3: Algorithmic Bias – Ensuring Fairness in Data Analytics
Adopting artificial intelligence has revealed that these technologies often display biases that frequently result in grave consequences for disadvantaged groups. These include harsher prison sentences and limited access to essential services like accommodation. There is increasing pressure on companies to make AI systems transparent and mitigate biases in their AI systems.
Subsection 3.1: Understanding Bias in Data
Unlike traditional computing engines, engineers “train” many types of AI systems on data instead of writing instructions that they follow. Therefore, AI systems rely on the data used to train them. Consequently, they will exhibit any biases in the training data, and companies must try to remove them. Companies should build diverse and representative data sets to train machine learning algorithms. They should also employ data cleaning technologies to remove biased or skewed data points. AI fairness tools are also available to monitor and mitigate bias during model training. Companies must also regularly audit data sets to detect and address hidden biases.
Subsection 3.2: Ethical Challenges of Big Data in AI Design
The wider community is increasingly aware of AI systems’ potential harmful effects and is pressuring companies to introduce them ethically. Therefore, they must make model decision-making transparent and ensure algorithms are free from unfair biases. They must also make explainable AI technologies that allow stakeholders to understand model decisions. Additionally, companies must demonstrate that they use fairness frameworks, like algorithmic impact assessments, to help evaluate ethical risk. Industries will need to collaborate on defining best practices for ethical AI design.
Subsection 3.3: Diversity in Data Science Teams
Biases in training data will affect the machine learning models they train. However, it also reflects the data science teams that built this data. Any biases in the team will also be present in the training data. Making data science teams diverse will help address this and equip the team to detect and mitigate algorithmic biases. Also, data science teams should collaborate with ethics experts to generate more equitable AI outcomes. Another action is for companies to use tools highlighting potential biases when hiring and forming data science teams. After hiring and forming teams, these teams should prioritize continuous education on bias and fairness. These measures will contribute to reducing the risk of unintentional biases in algorithms.
Subsection 3.4: Ethical Challenges of Big Data in Transparent Data Use
Regulatory authorities and the public at large are demanding far greater transparency. Therefore, companies must build public trust in their AI systems and be open about data sourcing and processing. Many individuals want to understand how AI models use their data; therefore, these systems must provide this transparency. Additionally, their AI models should include mechanisms to provide insights into their decision-making, as mentioned earlier. They should also build AI models on platforms that enable greater scrutiny. Many open-source platforms allow this.
Subsection 3.5: Regular Algorithm Audits
As mentioned, public and regulatory pressure is on companies to follow ethical AI practices. Companies will increasingly need to demonstrate that they are following ethical AI guidelines. Therefore, they must conduct regular audits to ensure that their AI models remain fair and unbiased over time. This includes collaboration with external auditors to demonstrate credibility that they address biases. Additionally, they should institutionalize these AI audits as part of their ethical data practices. They should utilize AI auditing tools that flag problematic patterns that point to potential biases. They should also continuously test these models to ensure no bias in the model after training with new data. These practices will help to guarantee that they will pass these audits.
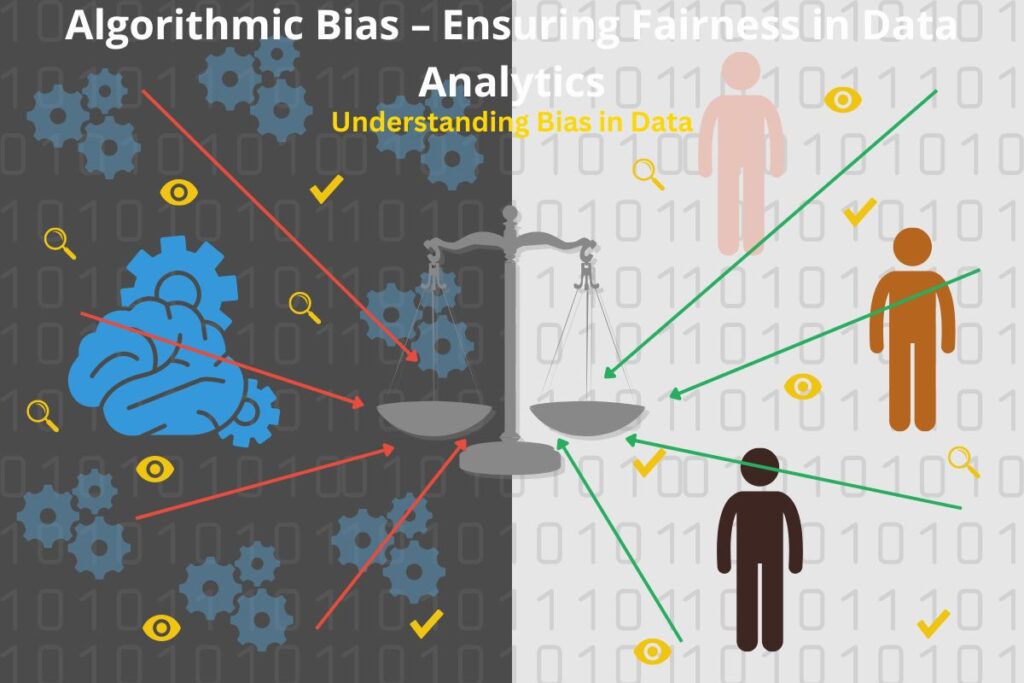
Section 4: Regulatory Compliance – Navigating Legal and Ethical Requirements
Subsection 4.1: GDPR and CCPA
Many regulatory authorities impose legal and ethical requirements on companies that use personal data. Europe and California have imposed the most demanding and far-reaching regulatory requirements in GDPR and CCPA, respectively. Therefore, technologies must support compliance with both global and local privacy regulations. Companies must design AI models to incorporate privacy to meet these regulatory requirements. They should also consider data compliance management systems that help automate adherence to legal and regulatory requirements. Companies should also regularly update compliance frameworks to align with evolving regulations.
Subsection 4.2: Sector-Specific Regulations
Regulatory requirements differ from industry to industry, each facing unique data regulations. For example, healthcare regulatory requirements differ from those of finance. Industries must consider automating compliance as it becomes more complex and demanding. They can implement regulatory technology (RegTech) solutions to automate compliance processes. Furthermore, automation will help companies stay updated on evolving regulations. AI systems compliance is sector-specific, and companies must tailor compliance systems to their industry. Another technology that companies should implement to adhere to regulatory environments is secure data sharing technologies.
Subsection 4.3: Data Protection Officer (DPO) Role
There is an increasingly harsh regulatory environment for data management where companies must adhere to data privacy and protection laws. Therefore, they should appoint a DPO to ensure adherence to demanding legal and regulatory data requirements. They must also empower their DPOs with the latest tools for ethical data management. Additionally, the DPO must ensure that data breach protocols are effectively implemented. Also, implementing blockchain can enhance the DPO’s ability to audit data flows. Another technology is AI-driven compliance tools that assist DPOs in monitoring and managing risks.
Subsection 4.4: Ethical Challenges of Big Data in Consent and Data Portability
Other onerous legal requirements now include consent management and data portability. We discussed consent earlier and showed that consent management technologies are crucial for ensuring legal compliance. Data portability allows users to transfer their data securely between service providers. Therefore, industries must adopt best practices for managing and transferring personal data. Additionally, companies that adopt technologies supporting consent and portability will earn customer trust. This includes building AI models that respect portability laws without compromising user privacy.
Subsection 4.5: Regulatory Fines and Penalties
With increasingly demanding legal and regulatory requirements, there are increasingly harsher fines and legal actions for non-compliance. Companies must implement organizational and technological solutions to ensure compliance and avoid costly penalties and grave legal actions. First, companies must collaborate or employ legal experts to ensure data practices remain within legal bounds. Next, companies should maintain a clear audit trail to demonstrate compliance. Companies should also purchase and implement compliance monitoring tools to help avoid legal and regulatory ramifications. Also, they should build or implement AI systems that integrate legal checks to ensure continuous regulatory adherence.
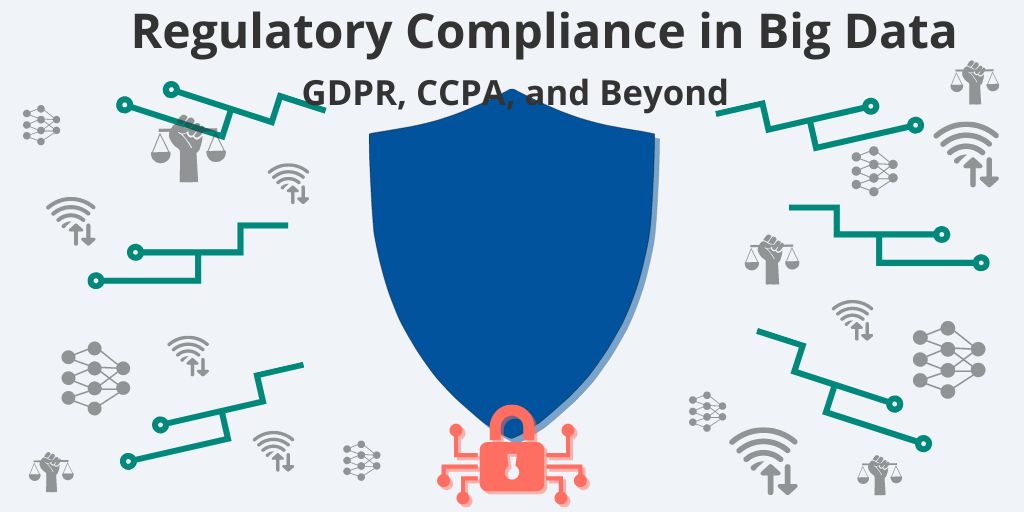
Section 5: Best Practices for Ethical Data Use
Companies are responsible for how their employees handle their data and must demonstrate good corporate citizenship. Growing public and regulatory pressure demands that companies handle their data ethically.
Subsection 5.1: Developing Ethical Guidelines
Companies and industry sectors should lead the establishment of ethical guidelines for data collection and use. This will help them win and maintain public trust. Additionally, they should involve diverse stakeholders in shaping these ethical data guidelines. Companies should also collaborate across industries to set common standards for ethical data use. Because technologies and challenges are evolving, companies should regularly update these guidelines. Companies should also consider utilizing technologies like AI ethics frameworks to provide guidance for fair data practices.
Subsection 5.2: Promoting Transparency
Another way for companies to build trust with consumers is to adopt transparency in data use. Transparency will also compel companies to enforce ethical data use within their operations. Companies can also reduce suspicion of their big data practices by allowing open communication on their data policies. They can further build trust through publicly available audits and reports. Companies should also invest in AI tools that explain data usage and decision-making to contribute to building transparency.
Subsection 5.3: Educating Teams on Ethics
Ethics in data usage require cooperation from all organization members and collaboration between ethicists and technologists. Training and education across the organization are the first steps in adopting an ethical data usage culture. This will empower teams to make more responsible decisions in data analysis. Training teams to understand the negative consequences of unethical data usage is a critical component. It includes the eventual impact on the organization they work for. Another component is AI ethics workshops that enable teams to recognize better and address potential data biases. This education must continue because teams must stay updated on new ethical standards and technologies.
Subsection 5.4: Engaging Consumers in Data Decisions
Consumer and community engagement in data usage decisions is a core component for adopting ethical data usage patterns. This will also foster trust in companies’ ethical data practices. Companies are responsible for giving consumers the most control over their data collection and use. They should also build AI models that respect consumer preferences when processing personal data. Additionally, companies should implement feedback loops that express concerns regarding data use, both technical and organizational implementations. Companies should also implement technologies that provide clear consent options to empower consumers to make informed choices.
Subsection 5.5: Implementing Privacy-Enhancing Technologies (PETs)
Technological solutions also help companies adopt ethical data usage practices. This includes PETs, which allow companies to analyze data while preserving individual privacy, which industries should adopt. PET technologies include federated learning, which ensures that data is processed without being centralized. They also include differential privacy methods to help maintain privacy in large-scale data analysis. Industries should build AI systems to incorporate PETs to minimize the risk of privacy breaches.
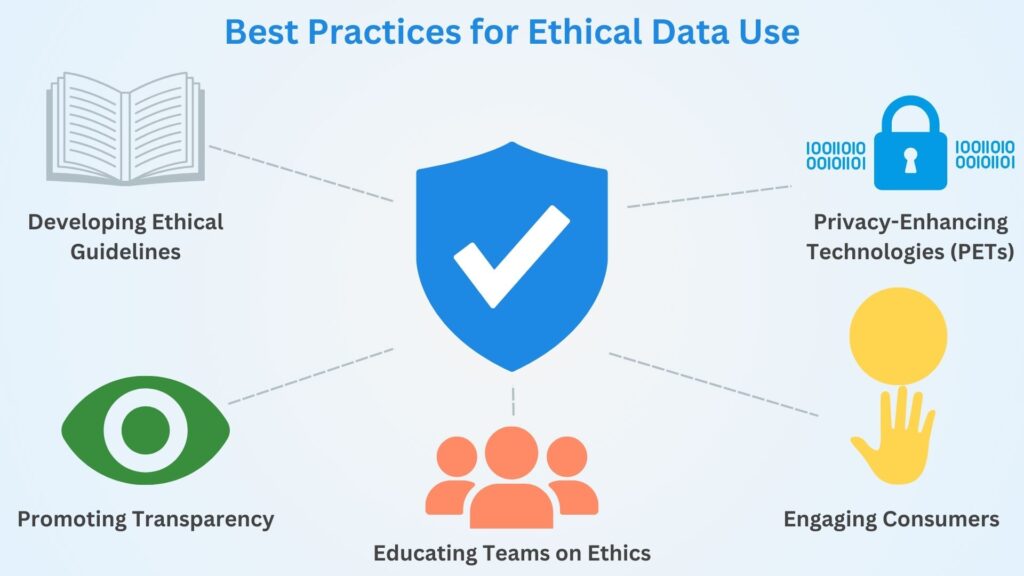
Conclusion: The Path to Ethical Big Data Practices
The rapid growth of big data presents opportunities and ethical challenges for many companies. All organizations must address these challenges through both technological and organizational solutions. Organizations adopting data-driven initiatives must make ethical considerations central to that effort. Companies implementing best practices and embracing transparency are better placed to ensure responsible data usage. Industries prioritizing ethical data usage can foster innovation while protecting individuals’ rights.