Processing large and complex datasets, known as Big Data, is transforming many industries. Big data applications in healthcare and finance are particularly noteworthy, as they enable better decision-making, improve service delivery, and unlock new growth opportunities. This technological advancement has significantly enhanced decision-making, personalized services, and operational efficiencies within these sectors.
Introduction to Big Data
Big data is an umbrella term that covers large and complex datasets and their processing. Its characteristics include volume, velocity, and variety. Big data also includes the necessary technologies and tools needed for processing these datasets along with its impact on society.
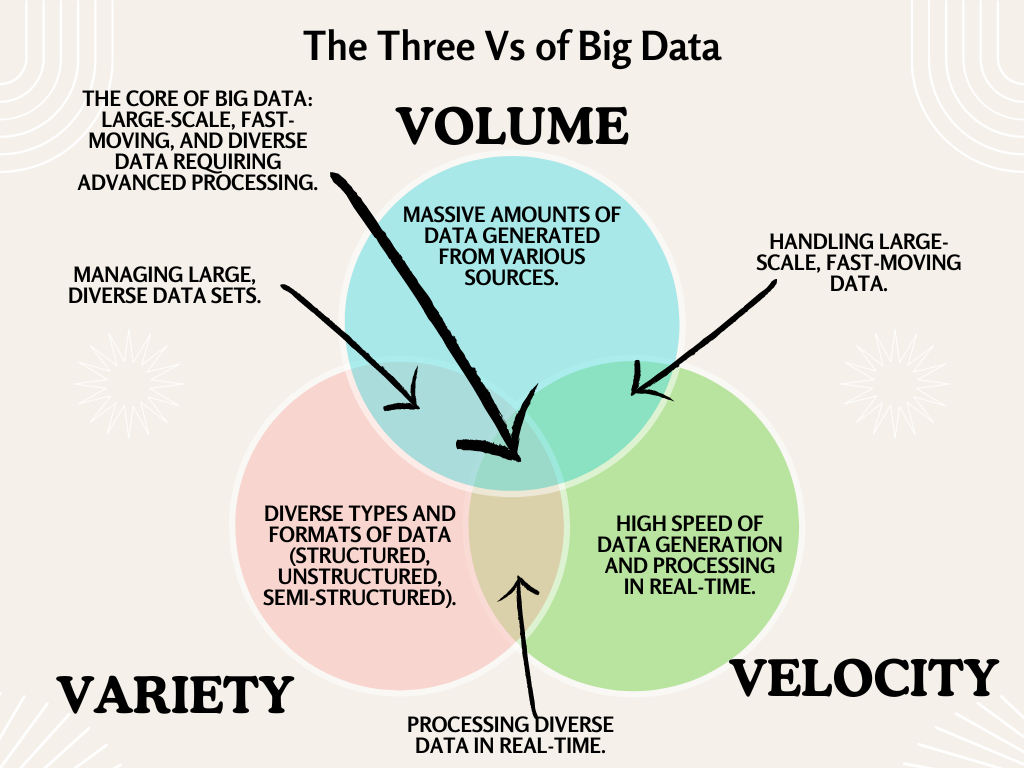
Origins and Evolution of Big Data
Hardware cost and performance improved exponentially, commoditizing it. This allowed distributed computing to address data proliferation. Distributed computing was able to handle extremely large data sets when open-source frameworks like Hadoop and MapReduce were introduced. It was at this time that big data became an independent field of study.
Relational Databases and the Internet Era
The introduction of relational database management systems (RDBMS) increased this rate of progress trajectory. RDBMS introduced structured data storage and querying, laying the foundation for modern data management. Internet proliferation caused digital data to explode. This resulted in a diverse range of data structures along with unstructured data and an ever-increasing volume. This stretched the ability of the current generation of data storage and analysis systems. Other developments that contributed to the proliferation of digital data included social media and data sensor technologies.
Distributed Computing and Open-Source Frameworks
Hardware cost and performance improved exponentially, commoditizing it. This allowed distributed computing to address data proliferation. Introducing open-source frameworks like Hadoop and MapReduce enabled distributed computing to handle extremely large data sets. It was at this time that big data became an independent field of study.
NoSQL Databases and Cloud Computing
Data proliferation also resulted in a proliferation of the diversity of data types. Another consequence was the need to process unstructured data. This necessitated the need for database technologies other than relational databases, commonly categorized as NoSQL databases. Their design enabled them to handle diverse data types that improved the scalability and flexibility of applications handling big data.
Cloud computing enables many organizations and individuals to utilize big data applications. It provides scalable and cost-efficient infrastructure for the storage, processing, and analytics of large datasets.
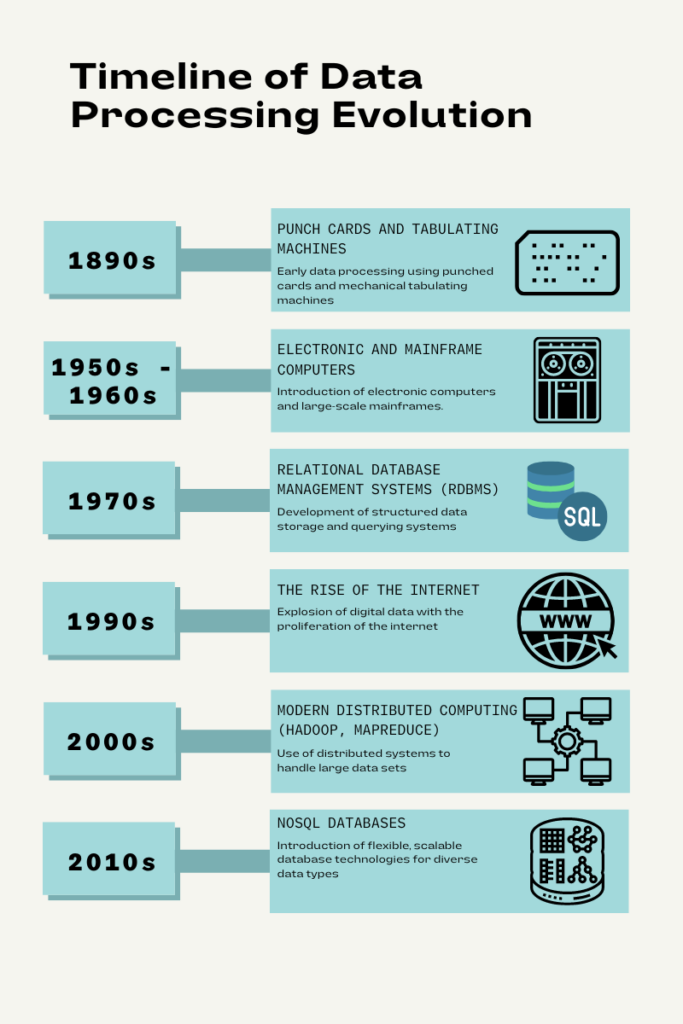
Future Trends and Ethical Considerations
Recent technological advances are beginning to disrupt big data even further and will have a profound impact on big data for many years to come. In summary, these include real-time analytics, integration of machine learning and artificial intelligence, and edge computing.
However, the impact of big data on both the individual and society is mixed, with privacy being a key example and the need for clear ethical guidelines in its use. New legal and regulatory frameworks will need to be developed to mitigate the harmful aspects of big data.
Big data is not only impactful but has transformed society in many profound ways.
Big Data: Transforming Industries Across Sectors
The impact of big data on business can not be understated. Big data is transforming many industries and the changes will be long-lasting. Big data is transforming many business activities. These include market analysis, customer insights, personalization, operational efficiency, risk management, predictive analytics, and performance tracking.
Driving Innovation with Big Data: Transforming Retail Industries
Market analysis is traditionally a data-driven area of business but the ability to process far greater magnitudes of data enhances the following areas. Large data sets can better identify emerging trends and opportunities in the marketplace, analyze competitor strategies and market positioning, and better forecast demand for products and services. A larger customer dataset allows a more accurate understanding of customer preferences and buying behaviors. All these insights feed into the ability to better optimize marketing strategies and campaigns.
Collective customer data can also be further utilized in finer and more accurate segmentation based on demographics, behaviors, and preferences whereas individual customer data can uncover customer lifecycle and purchasing patterns. These insights can allow businesses to better personalize marketing messages and offers to enhance engagement, thereby improving customer satisfaction and loyalty. Furthermore, businesses can better identify upselling and cross-selling opportunities. improving retail operations with big data allows businesses to forecast demand, manage inventory, and provide personalized customer experiences.
The article Smart Shopping: How Big Data is Personalizing the Retail Experience further explores improving retail operations with big data.
Personalization and Privacy Concerns
Personalization is a key opportunity for business that is enhanced by the insights provided by big data analysis of customer data in addition to marketing messages and promotions. Analysis of past purchases and web browsing history allows targeted product and service recommendations. Insights on user preferences allow for tailoring website content and user experience and managing personal interactions that enhance customer retention and lifetime value. By tracking customer preferences and analyzing purchasing patterns, companies are improving retail operations with big data, ensuring that products are readily available and tailored to consumer needs.
However, it is important to note that this will also result in privacy issues and other ethical considerations and there needs to be an ongoing discussion of business stewardship of personal data.
Operational Efficiency and Supply Chain Management
Alongside marketing and customer relationships, operational data is also an important area where big data is utilized to improve business operations in delivering products and services to customers. Another application of demand forecasting outlined above is to optimize inventory levels better and prevent stockouts. Insights derived from operational data enhance predictive maintenance and operation, identification of inefficiencies and bottlenecks, improved resource allocation, and workforce management. Also, supplier data allows insights that will optimize supply chain management further reducing costs and improving efficiency. Please explore Smart Manufacturing: Unlocking the Power of Big Data to understand better predictive maintenance using big data in manufacturing.
Risk Management and Predictive Analytics
Risk management can also be enhanced by utilizing market, customer, and operational data outlined above as well as financial data for the business. As mentioned at the start, predictive analysis and performance tracking are other areas, but there is an overlap with the areas discussed above and will not be further elaborated. Many organizations use Apache Spark for large-scale data processing and real-time predictive analytics in risk management. To explore its capabilities, check out our Apache Spark Big Data Analytics Guide.
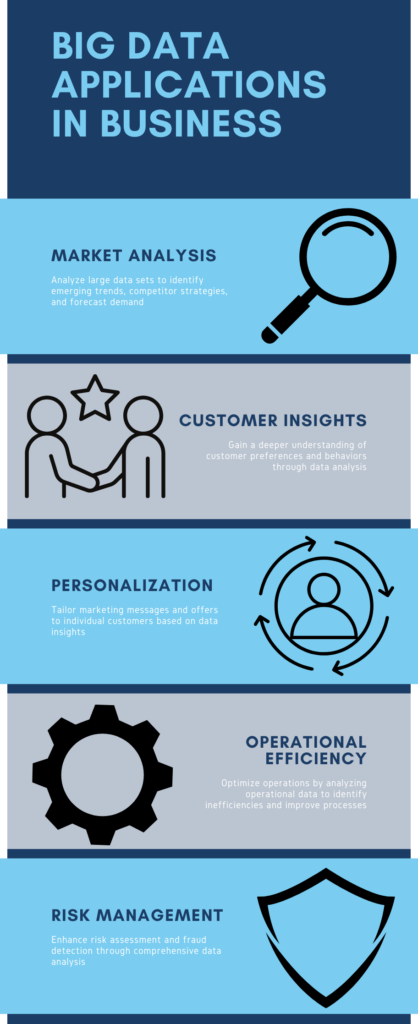
Big Data: Transforming Industries in Healthcare
How Big Data is Transforming Industries in Healthcare
Big data has the potential to revolutionize healthcare, especially in the area of surmounting healthcare costs, but is fraught with serious concerns around the security and management of sensitive patient data. Other key concerns are data ownership and algorithmic biases. Both the individual and the population are ripe for disruption in healthcare as well as medical research. There is also significant overlap in these areas and datasets apply to multiple different fields encompassed by healthcare.
Patient Care and Personalized Medicine
Healthcare ultimately is centered around the patient with its goal of optimizing patients’ health through a combination of prevention and cure as well as at an optimal cost. Datasets related to the patient, including patient history, provide insights into a range of patient care activities. Better tailoring of treatment plans and interventions can be achieved, and personalized medicine will allow prediction and prevention of adverse events. Potential risks and medical interactions are also better identifiable, which will improve patient safety. These will lead to better monitoring of patient progress and optimal care delivery, as well as better patient engagement.
Wearable Devices and Telemedicine
A developing area of patient data comes from wearable devices and sensors that can contribute to overall patient care as well as telemedicine that can significantly reduce health care costs. Real-time data from these sensors allows the monitoring of vital signs and health indicators as well as the detection of anomalies from normal health parameters, allowing early intervention. This can be extended to proactive healthcare interventions based on continuous monitoring and providing health coaching to patients so they are empowered to assume greater control of their health.
Clinical Data and Decision Support Systems
A key subset of patient data is clinical data, and decision support systems built around it will contribute to overall patient care. Data-driven insights will better assist healthcare providers in diagnosis and treatment decision-making, and integration with electronic health records (EHRs) will support evidence-based medicine. Decision-support systems will enable precision medicine and personalized treatment plans, as mentioned above, while data analytics can alert clinicians to potential medication errors, drug interactions, and adverse effects. Also, improvement of communication and interaction between multidisciplinary healthcare teams is achievable.
Population Health Management and Medical Research
Handling datasets that improve population health management will eventually lead to improved health care of the individual patient. Specifically, this is around disease prevention and better enabling early intervention due to insights into epidemiological trends as well as tracking disease outbreaks as well as identifying at-risk groups.
Medical research benefiting from big data analytics will also contribute to better health care for the individual patient. Big data raises modeling and simulation to a whole new level that will greatly enhance drug discovery and improve patient care. Other areas enhanced by large datasets include the identification of biomarkers for disease detection and diagnosis and genomic research. Also, institutions can share and analyze data that will facilitate greater collaboration.
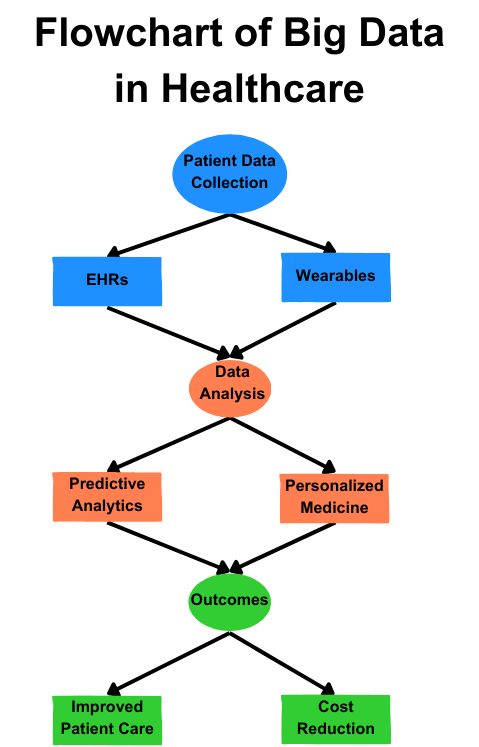
Big Data: Transforming Industries in Finance
Enhancing Financial Services with Big Data
Big data’s impact on business was discussed above, and its impact on different industries varies. However, the Finance industry is by nature data-driven and has always been an early adopter of data processing technologies. Big data applications in healthcare and finance enhances activities specific to the finance industry, including risk assessment, fraud detection, algorithmic trading, and compliance monitoring as well as other general business activities outlined earlier, including customer segmentation.
Risk Assessment and Fraud Detection
Risk assessment is the DNA of the finance industry, and its profitability is dependent on the reliability of risk assessments. Information that contributes to risk assessment includes financial histories, credit scores, and macroeconomic indicators. Firstly big data allows a far greater magnitude of the collection of raw data as well as faster processing for subsequent analysis and assessment, enabling it to be far more data-driven and reduction of uncertainty. This also includes a wider variety of data sources, including news, and social media. Big data also provides modeling of this data and can implement stress testing scenarios.
The finance industry by nature is a prime target for fraudulent activities and effective fraud detection is also essential to the industry’s profitability. Again big data allows the real-time collection of vast data from a great variety of data sources that provides far more signatures of fraudulent activities. It is then possible to utilize machine learning algorithms to detect fraudulent activities coupled with real-time monitoring of transactions. Another benefit is collaboration with law enforcement agencies on the detection and response to fraudulent activities.
Algorithmic Trading and Compliance Monitoring
The finance industry engages in trading to further boost its profits. Also, algorithmic trading is strongly data-driven big data enhancements, including analysis of much wider data sources. This includes news and social media to more accurately gauge market sentiment and investor behavior. Data analysis activities enhanced by big data include trading strategies due to big data analysis of market trends and patterns. Other activities include high-speed data processing for short-term trades and algorithm optimization.
Other considerations include compliance monitor and data stewardship. The finance industry, by nature, is responsible for highly sensitive data. Therefore, it is subject to strict regulatory requirements for managing this data. Also, regulatory authorities have placed legal requirements in place for the finance industry to have strong cybersecurity measures. These legal requirements will certainly become more stringent, as well as the proper use of sensitive data. The expansion of big data applications in healthcare and finance underscores the transformative power of data-driven decision-making. Therefore, it sets a new standard for operational excellence.
Big Data: Transforming Industries in Education
Spiraling costs plague education just like healthcare. However, because education is information-based, there are opportunities for disruption by big data to address some of the spiraling costs and inefficiencies.
Personalized Learning and Learning Analytics
It is also important to note that big data cannot address many factors, including teacher quality. It is important to recognize these limitations. Big data can enhance personalized learning, learning analytics, adaptive learning systems, student engagement, and predictive modeling. Big data can improve these fields where decisions are far more data-based and informed than previously. Also, big data analytic tools help to enhance data-based decision-making.
Adaptive Learning Systems and Resource Allocation
The ability to automate adaptive processes for learning materials, tutoring systems, and learning pathways and activities. This real-time automation will address many of the costs associated with education by reducing wasted time and resources. Other opportunities for cost reduction include reducing the need for redundant instruction and optimizing resource allocation for individual student needs. Further, big data can contribute to the empowerment of both students and teachers. Students can take ownership of their learning paths, whilst teachers can improve instruction through data-driven insights.
References
- This book provides a comprehensive overview of how big data is changing various aspects of society, including business, healthcare, and more.
- This book focuses on how businesses can leverage big data to improve decision-making, marketing, and operational efficiency.
- This book offers case studies from various industries, demonstrating how companies have successfully implemented big data analytics.
“Healthcare Analytics for Quality and Performance Improvement” by Trevor L. Strome
- This book provides insights into how big data analytics is transforming healthcare by improving patient care, operational efficiency, and decision-making.